Bayes factor vs P value
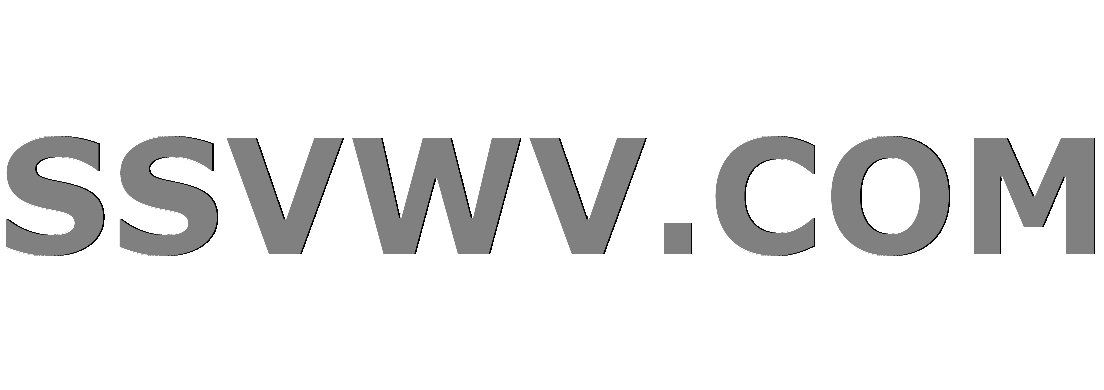
Multi tool use
.everyoneloves__top-leaderboard:empty,.everyoneloves__mid-leaderboard:empty,.everyoneloves__bot-mid-leaderboard:empty{ margin-bottom:0;
}
$begingroup$
I am trying to understand Bayes Factor (BF). I believe they are like likelihood ratio of 2 hypotheses. So if BF is 5, it means H1 is 5 times more likely than H0. And value of 3-10 indicates moderate evidence, while >10 indicates strong evidence.
However, for P-value, traditionally 0.05 is taken as cut-off. At this P value, H1/H0 likelihood should be 95/5 or 19.
So why a cut-off of >3 is taken for BF while a cut-off of >19 is taken for P values? These values are not anywhere close either.
I may be missing something very basic since I am a beginner in this area.
hypothesis-testing bayesian p-value
$endgroup$
add a comment |
$begingroup$
I am trying to understand Bayes Factor (BF). I believe they are like likelihood ratio of 2 hypotheses. So if BF is 5, it means H1 is 5 times more likely than H0. And value of 3-10 indicates moderate evidence, while >10 indicates strong evidence.
However, for P-value, traditionally 0.05 is taken as cut-off. At this P value, H1/H0 likelihood should be 95/5 or 19.
So why a cut-off of >3 is taken for BF while a cut-off of >19 is taken for P values? These values are not anywhere close either.
I may be missing something very basic since I am a beginner in this area.
hypothesis-testing bayesian p-value
$endgroup$
add a comment |
$begingroup$
I am trying to understand Bayes Factor (BF). I believe they are like likelihood ratio of 2 hypotheses. So if BF is 5, it means H1 is 5 times more likely than H0. And value of 3-10 indicates moderate evidence, while >10 indicates strong evidence.
However, for P-value, traditionally 0.05 is taken as cut-off. At this P value, H1/H0 likelihood should be 95/5 or 19.
So why a cut-off of >3 is taken for BF while a cut-off of >19 is taken for P values? These values are not anywhere close either.
I may be missing something very basic since I am a beginner in this area.
hypothesis-testing bayesian p-value
$endgroup$
I am trying to understand Bayes Factor (BF). I believe they are like likelihood ratio of 2 hypotheses. So if BF is 5, it means H1 is 5 times more likely than H0. And value of 3-10 indicates moderate evidence, while >10 indicates strong evidence.
However, for P-value, traditionally 0.05 is taken as cut-off. At this P value, H1/H0 likelihood should be 95/5 or 19.
So why a cut-off of >3 is taken for BF while a cut-off of >19 is taken for P values? These values are not anywhere close either.
I may be missing something very basic since I am a beginner in this area.
hypothesis-testing bayesian p-value
hypothesis-testing bayesian p-value
edited 2 hours ago
rnso
asked 3 hours ago
rnsornso
4,067103168
4,067103168
add a comment |
add a comment |
2 Answers
2
active
oldest
votes
$begingroup$
A few things:
The BF gives you evidence in favor of a hypothesis, while a frequentist hypothesis test gives you evidence against a (null) hypothesis. So it's kind of "apples to oranges."
These two procedures, despite the difference in interpretations, may lead to different decisions. For example, a BF might reject while a frequentist hypothesis test doesn't, or vice versa. This problem is often referred to as the Jeffreys-Lindley's paradox. There have been many posts on this site about this; see e.g. here, and here.
"At this P value, H1/H0 likelihood should be 95/5 or 19." No, this isn't true because, roughly $p(y mid H_1) neq 1- p(y mid H_0)$. Computing a p-value and performing a frequentist test, at a minimum, does not require you to have any idea about $p(y mid H_1)$. Also, p-values are often integrals/sums of densities/pmfs, while a BF doesn't integrate over the data sample space.
$endgroup$
$begingroup$
Thanks for your insight. However, if evidence in favor of a hypothesis isapple
, I think evidence for alternate hypothesis can beinverted apple
but notorange
! Also, what would you say is approximate Bayes Factor value corresponding to P=0.05?
$endgroup$
– rnso
1 hour ago
add a comment |
$begingroup$
The Bayes factor $B_{01}$ can be turned into a probability under equal weights as
$$P_{01}=frac{1}{1+frac{1}{large B_{01}}}$$but this does not make them comparable with a $p$-value since
$P_{01}$ is a probability in the parameter space, not in the sampling space- its value and range depend on the choice of the prior measure, they are thus relative rather than absolute
- both $B_{01}$ and $P_{01}$ contain a penalty for complexity (Occam's razor) by integrating out over the parameter space
If you wish to consider a Bayesian equivalent to the $p$-value, the posterior predictive $p$-value (Meng, 1994) should be investigated
$$Q_{01}=mathbb P(B_{01}(X)le B_{01}(x^text{obs}))$$
where $x^text{obs}$ denotes the observation and $X$ is distributed from the posterior predictive
$$Xsim int_Theta f(x|theta) pi(theta|x^text{obs}),text{d}theta$$
but this does not imply that the same "default" criteria for rejection and significance should apply to this object.
$endgroup$
add a comment |
Your Answer
StackExchange.ready(function() {
var channelOptions = {
tags: "".split(" "),
id: "65"
};
initTagRenderer("".split(" "), "".split(" "), channelOptions);
StackExchange.using("externalEditor", function() {
// Have to fire editor after snippets, if snippets enabled
if (StackExchange.settings.snippets.snippetsEnabled) {
StackExchange.using("snippets", function() {
createEditor();
});
}
else {
createEditor();
}
});
function createEditor() {
StackExchange.prepareEditor({
heartbeatType: 'answer',
autoActivateHeartbeat: false,
convertImagesToLinks: false,
noModals: true,
showLowRepImageUploadWarning: true,
reputationToPostImages: null,
bindNavPrevention: true,
postfix: "",
imageUploader: {
brandingHtml: "Powered by u003ca class="icon-imgur-white" href="https://imgur.com/"u003eu003c/au003e",
contentPolicyHtml: "User contributions licensed under u003ca href="https://creativecommons.org/licenses/by-sa/3.0/"u003ecc by-sa 3.0 with attribution requiredu003c/au003e u003ca href="https://stackoverflow.com/legal/content-policy"u003e(content policy)u003c/au003e",
allowUrls: true
},
onDemand: true,
discardSelector: ".discard-answer"
,immediatelyShowMarkdownHelp:true
});
}
});
Sign up or log in
StackExchange.ready(function () {
StackExchange.helpers.onClickDraftSave('#login-link');
});
Sign up using Google
Sign up using Facebook
Sign up using Email and Password
Post as a guest
Required, but never shown
StackExchange.ready(
function () {
StackExchange.openid.initPostLogin('.new-post-login', 'https%3a%2f%2fstats.stackexchange.com%2fquestions%2f404933%2fbayes-factor-vs-p-value%23new-answer', 'question_page');
}
);
Post as a guest
Required, but never shown
2 Answers
2
active
oldest
votes
2 Answers
2
active
oldest
votes
active
oldest
votes
active
oldest
votes
$begingroup$
A few things:
The BF gives you evidence in favor of a hypothesis, while a frequentist hypothesis test gives you evidence against a (null) hypothesis. So it's kind of "apples to oranges."
These two procedures, despite the difference in interpretations, may lead to different decisions. For example, a BF might reject while a frequentist hypothesis test doesn't, or vice versa. This problem is often referred to as the Jeffreys-Lindley's paradox. There have been many posts on this site about this; see e.g. here, and here.
"At this P value, H1/H0 likelihood should be 95/5 or 19." No, this isn't true because, roughly $p(y mid H_1) neq 1- p(y mid H_0)$. Computing a p-value and performing a frequentist test, at a minimum, does not require you to have any idea about $p(y mid H_1)$. Also, p-values are often integrals/sums of densities/pmfs, while a BF doesn't integrate over the data sample space.
$endgroup$
$begingroup$
Thanks for your insight. However, if evidence in favor of a hypothesis isapple
, I think evidence for alternate hypothesis can beinverted apple
but notorange
! Also, what would you say is approximate Bayes Factor value corresponding to P=0.05?
$endgroup$
– rnso
1 hour ago
add a comment |
$begingroup$
A few things:
The BF gives you evidence in favor of a hypothesis, while a frequentist hypothesis test gives you evidence against a (null) hypothesis. So it's kind of "apples to oranges."
These two procedures, despite the difference in interpretations, may lead to different decisions. For example, a BF might reject while a frequentist hypothesis test doesn't, or vice versa. This problem is often referred to as the Jeffreys-Lindley's paradox. There have been many posts on this site about this; see e.g. here, and here.
"At this P value, H1/H0 likelihood should be 95/5 or 19." No, this isn't true because, roughly $p(y mid H_1) neq 1- p(y mid H_0)$. Computing a p-value and performing a frequentist test, at a minimum, does not require you to have any idea about $p(y mid H_1)$. Also, p-values are often integrals/sums of densities/pmfs, while a BF doesn't integrate over the data sample space.
$endgroup$
$begingroup$
Thanks for your insight. However, if evidence in favor of a hypothesis isapple
, I think evidence for alternate hypothesis can beinverted apple
but notorange
! Also, what would you say is approximate Bayes Factor value corresponding to P=0.05?
$endgroup$
– rnso
1 hour ago
add a comment |
$begingroup$
A few things:
The BF gives you evidence in favor of a hypothesis, while a frequentist hypothesis test gives you evidence against a (null) hypothesis. So it's kind of "apples to oranges."
These two procedures, despite the difference in interpretations, may lead to different decisions. For example, a BF might reject while a frequentist hypothesis test doesn't, or vice versa. This problem is often referred to as the Jeffreys-Lindley's paradox. There have been many posts on this site about this; see e.g. here, and here.
"At this P value, H1/H0 likelihood should be 95/5 or 19." No, this isn't true because, roughly $p(y mid H_1) neq 1- p(y mid H_0)$. Computing a p-value and performing a frequentist test, at a minimum, does not require you to have any idea about $p(y mid H_1)$. Also, p-values are often integrals/sums of densities/pmfs, while a BF doesn't integrate over the data sample space.
$endgroup$
A few things:
The BF gives you evidence in favor of a hypothesis, while a frequentist hypothesis test gives you evidence against a (null) hypothesis. So it's kind of "apples to oranges."
These two procedures, despite the difference in interpretations, may lead to different decisions. For example, a BF might reject while a frequentist hypothesis test doesn't, or vice versa. This problem is often referred to as the Jeffreys-Lindley's paradox. There have been many posts on this site about this; see e.g. here, and here.
"At this P value, H1/H0 likelihood should be 95/5 or 19." No, this isn't true because, roughly $p(y mid H_1) neq 1- p(y mid H_0)$. Computing a p-value and performing a frequentist test, at a minimum, does not require you to have any idea about $p(y mid H_1)$. Also, p-values are often integrals/sums of densities/pmfs, while a BF doesn't integrate over the data sample space.
edited 17 mins ago


Xi'an
59.8k897369
59.8k897369
answered 2 hours ago
TaylorTaylor
12.7k21946
12.7k21946
$begingroup$
Thanks for your insight. However, if evidence in favor of a hypothesis isapple
, I think evidence for alternate hypothesis can beinverted apple
but notorange
! Also, what would you say is approximate Bayes Factor value corresponding to P=0.05?
$endgroup$
– rnso
1 hour ago
add a comment |
$begingroup$
Thanks for your insight. However, if evidence in favor of a hypothesis isapple
, I think evidence for alternate hypothesis can beinverted apple
but notorange
! Also, what would you say is approximate Bayes Factor value corresponding to P=0.05?
$endgroup$
– rnso
1 hour ago
$begingroup$
Thanks for your insight. However, if evidence in favor of a hypothesis is
apple
, I think evidence for alternate hypothesis can be inverted apple
but not orange
! Also, what would you say is approximate Bayes Factor value corresponding to P=0.05?$endgroup$
– rnso
1 hour ago
$begingroup$
Thanks for your insight. However, if evidence in favor of a hypothesis is
apple
, I think evidence for alternate hypothesis can be inverted apple
but not orange
! Also, what would you say is approximate Bayes Factor value corresponding to P=0.05?$endgroup$
– rnso
1 hour ago
add a comment |
$begingroup$
The Bayes factor $B_{01}$ can be turned into a probability under equal weights as
$$P_{01}=frac{1}{1+frac{1}{large B_{01}}}$$but this does not make them comparable with a $p$-value since
$P_{01}$ is a probability in the parameter space, not in the sampling space- its value and range depend on the choice of the prior measure, they are thus relative rather than absolute
- both $B_{01}$ and $P_{01}$ contain a penalty for complexity (Occam's razor) by integrating out over the parameter space
If you wish to consider a Bayesian equivalent to the $p$-value, the posterior predictive $p$-value (Meng, 1994) should be investigated
$$Q_{01}=mathbb P(B_{01}(X)le B_{01}(x^text{obs}))$$
where $x^text{obs}$ denotes the observation and $X$ is distributed from the posterior predictive
$$Xsim int_Theta f(x|theta) pi(theta|x^text{obs}),text{d}theta$$
but this does not imply that the same "default" criteria for rejection and significance should apply to this object.
$endgroup$
add a comment |
$begingroup$
The Bayes factor $B_{01}$ can be turned into a probability under equal weights as
$$P_{01}=frac{1}{1+frac{1}{large B_{01}}}$$but this does not make them comparable with a $p$-value since
$P_{01}$ is a probability in the parameter space, not in the sampling space- its value and range depend on the choice of the prior measure, they are thus relative rather than absolute
- both $B_{01}$ and $P_{01}$ contain a penalty for complexity (Occam's razor) by integrating out over the parameter space
If you wish to consider a Bayesian equivalent to the $p$-value, the posterior predictive $p$-value (Meng, 1994) should be investigated
$$Q_{01}=mathbb P(B_{01}(X)le B_{01}(x^text{obs}))$$
where $x^text{obs}$ denotes the observation and $X$ is distributed from the posterior predictive
$$Xsim int_Theta f(x|theta) pi(theta|x^text{obs}),text{d}theta$$
but this does not imply that the same "default" criteria for rejection and significance should apply to this object.
$endgroup$
add a comment |
$begingroup$
The Bayes factor $B_{01}$ can be turned into a probability under equal weights as
$$P_{01}=frac{1}{1+frac{1}{large B_{01}}}$$but this does not make them comparable with a $p$-value since
$P_{01}$ is a probability in the parameter space, not in the sampling space- its value and range depend on the choice of the prior measure, they are thus relative rather than absolute
- both $B_{01}$ and $P_{01}$ contain a penalty for complexity (Occam's razor) by integrating out over the parameter space
If you wish to consider a Bayesian equivalent to the $p$-value, the posterior predictive $p$-value (Meng, 1994) should be investigated
$$Q_{01}=mathbb P(B_{01}(X)le B_{01}(x^text{obs}))$$
where $x^text{obs}$ denotes the observation and $X$ is distributed from the posterior predictive
$$Xsim int_Theta f(x|theta) pi(theta|x^text{obs}),text{d}theta$$
but this does not imply that the same "default" criteria for rejection and significance should apply to this object.
$endgroup$
The Bayes factor $B_{01}$ can be turned into a probability under equal weights as
$$P_{01}=frac{1}{1+frac{1}{large B_{01}}}$$but this does not make them comparable with a $p$-value since
$P_{01}$ is a probability in the parameter space, not in the sampling space- its value and range depend on the choice of the prior measure, they are thus relative rather than absolute
- both $B_{01}$ and $P_{01}$ contain a penalty for complexity (Occam's razor) by integrating out over the parameter space
If you wish to consider a Bayesian equivalent to the $p$-value, the posterior predictive $p$-value (Meng, 1994) should be investigated
$$Q_{01}=mathbb P(B_{01}(X)le B_{01}(x^text{obs}))$$
where $x^text{obs}$ denotes the observation and $X$ is distributed from the posterior predictive
$$Xsim int_Theta f(x|theta) pi(theta|x^text{obs}),text{d}theta$$
but this does not imply that the same "default" criteria for rejection and significance should apply to this object.
answered 5 mins ago


Xi'anXi'an
59.8k897369
59.8k897369
add a comment |
add a comment |
Thanks for contributing an answer to Cross Validated!
- Please be sure to answer the question. Provide details and share your research!
But avoid …
- Asking for help, clarification, or responding to other answers.
- Making statements based on opinion; back them up with references or personal experience.
Use MathJax to format equations. MathJax reference.
To learn more, see our tips on writing great answers.
Sign up or log in
StackExchange.ready(function () {
StackExchange.helpers.onClickDraftSave('#login-link');
});
Sign up using Google
Sign up using Facebook
Sign up using Email and Password
Post as a guest
Required, but never shown
StackExchange.ready(
function () {
StackExchange.openid.initPostLogin('.new-post-login', 'https%3a%2f%2fstats.stackexchange.com%2fquestions%2f404933%2fbayes-factor-vs-p-value%23new-answer', 'question_page');
}
);
Post as a guest
Required, but never shown
Sign up or log in
StackExchange.ready(function () {
StackExchange.helpers.onClickDraftSave('#login-link');
});
Sign up using Google
Sign up using Facebook
Sign up using Email and Password
Post as a guest
Required, but never shown
Sign up or log in
StackExchange.ready(function () {
StackExchange.helpers.onClickDraftSave('#login-link');
});
Sign up using Google
Sign up using Facebook
Sign up using Email and Password
Post as a guest
Required, but never shown
Sign up or log in
StackExchange.ready(function () {
StackExchange.helpers.onClickDraftSave('#login-link');
});
Sign up using Google
Sign up using Facebook
Sign up using Email and Password
Sign up using Google
Sign up using Facebook
Sign up using Email and Password
Post as a guest
Required, but never shown
Required, but never shown
Required, but never shown
Required, but never shown
Required, but never shown
Required, but never shown
Required, but never shown
Required, but never shown
Required, but never shown
2SOD67vnn,9Wp8HHfW4MixGkQ,T8gl44,9BDE4,8EaOJK3TAoL qSxFvtbMat,sBFJaNLXoN3Yx